The Multivariate Normal Distribution#
import torch
from torch.distributions import Normal, MultivariateNormal, Wishart
torch.manual_seed(305)
import matplotlib.pyplot as plt
import seaborn as sns
sns.set_context("notebook")
The Generative Story#
Start with a vector of standard normal random variates, \(\mathbf{z} = [z_1, \ldots, z_D]^\top\) where \(z_d {\sim} \mathcal{N}(0, 1)\).
This is a \(D\)-dimensional random variable, but not a very interesting one. All the coordinates are independent! The joint density is,
What do the contours of this joint density look like in \(D=2\) dimensions?
z1, z2 = torch.meshgrid(torch.linspace(-4, 4, 50),
torch.linspace(-4, 4, 50))
logpdf = Normal(0, 1).log_prob(z1) + Normal(0, 1).log_prob(z2)
plt.contour(z1, z2, logpdf)
plt.xlabel("$z_1$")
plt.ylabel("$z_2$")
plt.gca().set_aspect(1)
/opt/hostedtoolcache/Python/3.9.17/x64/lib/python3.9/site-packages/torch/functional.py:504: UserWarning: torch.meshgrid: in an upcoming release, it will be required to pass the indexing argument. (Triggered internally at ../aten/src/ATen/native/TensorShape.cpp:3483.)
return _VF.meshgrid(tensors, **kwargs) # type: ignore[attr-defined]
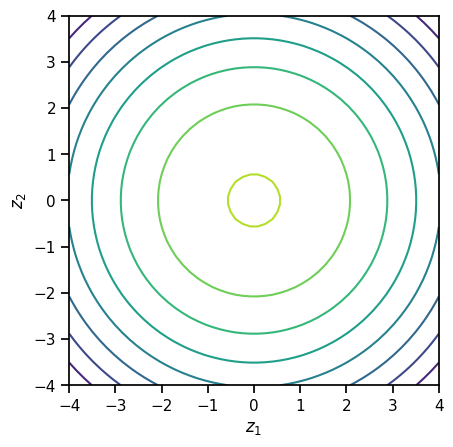
We can obtain more interesting joint distributions by transforming this random vector.
For example, let \(U\) be an orthogonal \(D\times D\) matrix and \(\Lambda = \mathrm{diag}([\lambda_1, \ldots, \lambda_D])\) with \(\lambda_d > 0\). Define the linearly transformed random variable \(x = U \Lambda^{\frac{1}{2}} z\).
# Make an orthogonal 2 x 2 matrix
theta1 = torch.tensor(torch.pi / 4)
u1 = torch.tensor([torch.cos(theta1), torch.sin(theta1)])
theta2 = theta1 + torch.pi / 2
u2 = torch.tensor([torch.cos(theta2), torch.sin(theta2)])
U = torch.column_stack([u1, u2])
# choose two eigenvales
lmbda1 = torch.tensor(2.0**2)
lmbda2 = torch.tensor(0.5**2)
Lmbda = torch.tensor([lmbda1, lmbda2])
# pick one isocontour in z space and transform it to x coordinates
zs = torch.column_stack([
torch.cos(torch.linspace(0, 2 * torch.pi, 50)),
torch.sin(torch.linspace(0, 2 * torch.pi, 50))
])
xs = (zs * torch.sqrt(Lmbda)) @ U.T
# Plot the new basis
plt.plot(xs[:, 0], xs[:, 1], ':')
plt.arrow(0, 0, torch.sqrt(lmbda1) * u1[0], torch.sqrt(lmbda1) * u1[1],
color='k', head_width=0.2)
plt.text(torch.sqrt(lmbda1) * u1[0], torch.sqrt(lmbda1) * u1[1] + .4,
"$\sqrt{\lambda_1} u_1$")
plt.arrow(0, 0, torch.sqrt(lmbda2) * u2[0], torch.sqrt(lmbda2) * u2[1],
color='k', head_width=0.2)
plt.text(torch.sqrt(lmbda2) * u2[0] - 1.5, torch.sqrt(lmbda2) * u2[1],
"$\sqrt{\lambda_2} u_2$")
plt.xlim(-3, 3)
plt.ylim(-3, 3)
plt.xlabel("$x_1$")
plt.ylabel("$x_2$")
plt.gca().set_aspect(1)
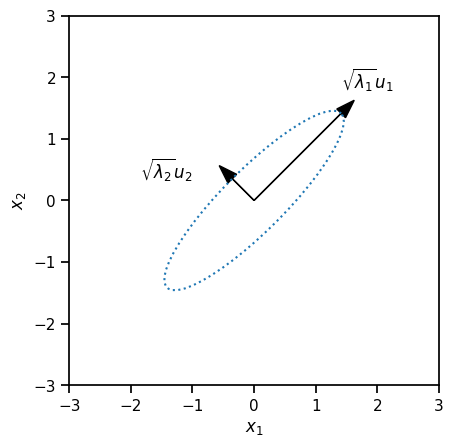
Write a function to visualize covariance matrices#
def plot_cov(Sigma, mu=None, ax=None, **kwargs):
"""
Simple function to visualize a covariance matrix.
"""
# Set the mean to zero if not given
D = Sigma.shape[-1]
mu = torch.zeros(D,) if mu is None else mu
# Compute the eigendecomposition
Lmbda, U = torch.linalg.eigh(Sigma)
# Find one isocontour
zs = torch.column_stack([
torch.cos(torch.linspace(0, 2 * torch.pi, 50)),
torch.sin(torch.linspace(0, 2 * torch.pi, 50))
])
xs = (zs * torch.sqrt(Lmbda)) @ U.T
# plot the isocontour
ax = plt.axes(aspect=1) if ax is None else ax
ax.plot(mu[0] + xs[:, 0], mu[1] + xs[:, 1], **kwargs)
Draw many samples from a Wishart distribution and plot their inverses#
nu0 = torch.tensor(4.)
Lmbda0 = torch.eye(2) / nu0
Lmbdas = Wishart(nu0, covariance_matrix=Lmbda0).sample(sample_shape=(5, 5))
fig, axs = plt.subplots(5, 5, figsize=(8, 8), sharex=True, sharey=True)
for i in range(5):
for j in range(5):
plot_cov(torch.inverse(Lmbdas[i, j]), ax=axs[i, j])
plot_cov(torch.eye(2), ax=axs[i, j], color='k')
axs[i, j].set_xlim(-4, 4)
axs[i, j].set_ylim(-4, 4)
axs[i, j].set_aspect(1)
for i in range(5):
axs[i, 0].set_ylabel("$x_2$")
axs[-1, i].set_xlabel("$x_1$")
/opt/hostedtoolcache/Python/3.9.17/x64/lib/python3.9/site-packages/torch/distributions/wishart.py:253: UserWarning: Singular sample detected.
warnings.warn("Singular sample detected.")
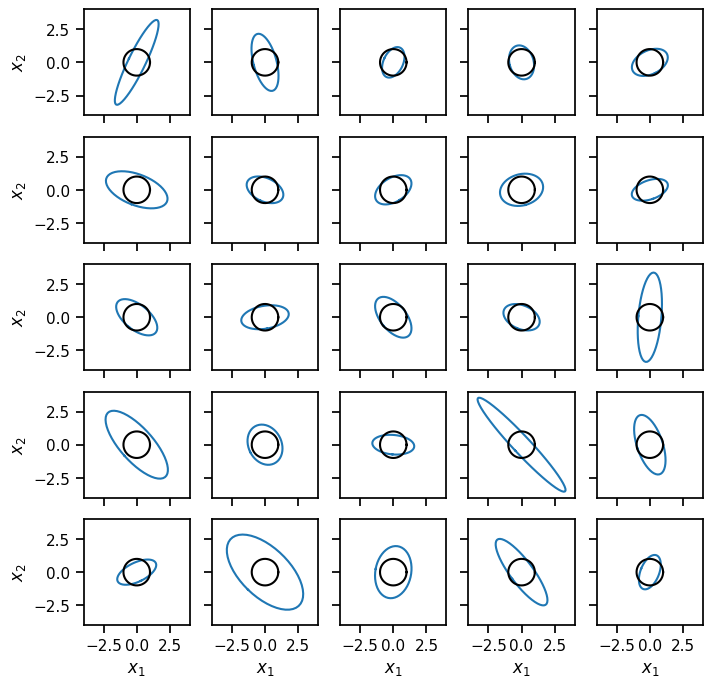
Bayesian inference with unknown precision#
The Wishart distribution is a conjugate prior for the precision of a multivariate normal distribution.
Then, letting \(\eta = (\mu, \nu_0, \Lambda_0)\),
We recognize this as yet another Wishart distribution,
where
# Sample data from a Gaussian with identity covariance (and precision)
torch.manual_seed(305)
N = 10
x = MultivariateNormal(torch.zeros(2), torch.eye(2)).sample((N,))
# Set a weak prior
nu_0 = torch.tensor(1.0)
Lmbda_0 = torch.eye(2)
# Compute the posterior distribution over the precision under a Wishart prior,
# assuming the mean is known to be zero
nu_N = nu_0 + N
Lmbda_N = torch.inverse(torch.inverse(Lmbda_0) + x.T @ x)
posterior = Wishart(nu_N, Lmbda_N)
# Plot posterior samples of the *covariance* (i.e. inverse precision)
precision_samples = posterior.sample((10,))
covariance_samples = torch.inverse(precision_samples)
ax = plt.axes(aspect=1)
for Sigma in covariance_samples:
plot_cov(Sigma, ax=ax, color='r', alpha=0.5)
plt.plot(x[:, 0], x[:, 1], 'ko', markersize=6)
plt.xlim(-4, 4)
plt.ylim(-4, 4)
plt.xlabel("$x_1$")
plt.ylabel("$x_2$")
Text(0, 0.5, '$x_2$')
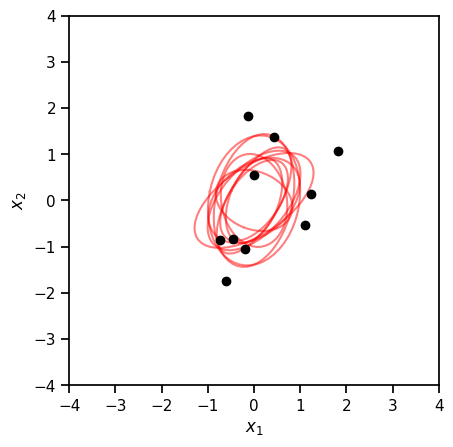
Bayesian Inference with Unknown Mean and Precision#
What if both the mean and the precision are unknown? Then a normal-Wishart prior is conjugate with the multivariate normal likelihood. We say
if
Under a multivariate Gaussian likelihood,
Then, the posterior on the parameters is another normal-Wishart distribution with parameters
Next, we’ll compute the posterior of \(\boldsymbol{\mu}\) and \(\boldsymbol{\Lambda}\) and visualize it.
# Set a weak prior
nu_0 = torch.tensor(1.0)
Lmbda_0 = torch.eye(2)
kappa_0 = torch.tensor(1.0)
mu_0 = torch.zeros((2,))
# Compute the posterior distribution over the mean and precision under a
# normal-Wishart prior with the hyperparameters above.
nu_N = nu_0 + N
kappa_N = kappa_0 + N
mu_N = 1/kappa_N * (kappa_0 * mu_0 + x.sum(axis=0))
Lmbda_N = torch.inverse(torch.inverse(Lmbda_0)
+ kappa_0 * torch.outer(mu_0, mu_0)
+ x.T @ x
- kappa_N * torch.outer(mu_N, mu_N))
# Sample the posterior
posterior_Lambda = Wishart(nu_N, Lmbda_N)
Lambda_samples = posterior.sample((100,))
Sigma_samples = torch.inverse(Lambda_samples)
posterior_mu = MultivariateNormal(mu_N,
precision_matrix=Lambda_samples * kappa_N)
mu_samples = posterior_mu.sample()
# Plot posterior samples of the Gaussian parameters
ax = plt.axes(aspect=1)
for i, (mu, Sigma) in enumerate(zip(mu_samples[:10], Sigma_samples[:10])):
plot_cov(Sigma, ax=ax, color='r', alpha=0.5,
label='$\Sigma$ samples' if i == 0 else None)
plt.plot(mu[0], mu[1], 'ro', markersize=3, mec='k',
label='$\mu$ samples' if i == 0 else None)
plt.plot(x[:, 0], x[:, 1], 'ko', markersize=6, label='data')
plt.xlim(-4, 4)
plt.ylim(-4, 4)
plt.xlabel("$x_1$")
plt.ylabel("$x_2$")
plt.legend()
<matplotlib.legend.Legend at 0x7fea840677c0>
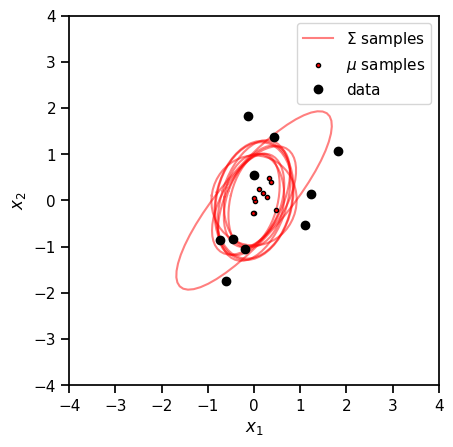
Recap#
This notebook introduced the MultivariateNormal
and Wishart
distributions. We gave examples of how you can visualize 2 dimensional covariance matrices (and inverse precision matrices) as ellipses in \(\mathbb{R}^2\). This is a helpful way of visualizing prior distributions, like the Wishart distribution.
A couple of notes:
PyTorch does not currently have an inverse Wishart distribution object. In theory, you could implement one as a
TransformedDistribution
, but you would need to also write aMatrixInverseTransform
object. None of this is particularly hard, but it was more than we chose to do for this demo.In practice, many practitioners suggest using the
LKJCholesky
distribution as a prior on covariance matrices (technically, their square roots). It doesn’t have as simple a closed form update as the Wishart or inverse Wishart, but it is amenable to other inference techniques that we’ll discuss later in the course.